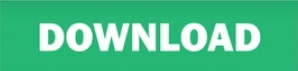
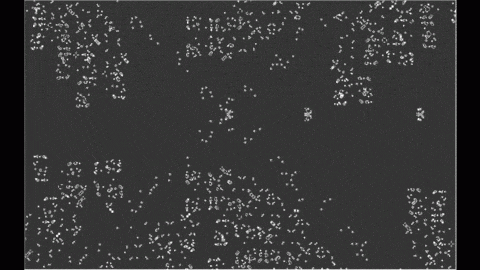
Luckily there are plenty of implementations available online, such as the following from. The first thing to do is define a function that takes a game board as input and returns the next state. It’s probably overkill for Conway’s Game, but it’s still pretty interesting to see how it performs. Why do this? Mostly for fun, and to learn a little bit about convolutional neural networks. The rules continue to be applied repeatedly to create further generations. Each generation is a pure function of the preceding one. The first generation is created by applying the above rules simultaneously to every cell in the seed births and deaths occur simultaneously, and the discrete moment at which this happens is sometimes called a tick. The initial pattern constitutes the seed of the system. Any dead cell with exactly three live neighbors becomes a live cell, as if by reproduction.Any live cell with more than three live neighbors dies, as if by overpopulation.Any live cell with two or three live neighbors lives on to the next generation.Any live cell with fewer than two live neighbors dies, as if by underpopulation.At each step in time, the following transitions occur: Every cell interacts with its eight neighbours, which are the cells that are horizontally, vertically, or diagonally adjacent. The universe of the Game of Life is an infinite, two-dimensional orthogonal grid of square cells, each of which is in one of two possible states, alive or dead, (or populated and unpopulated, respectively). Speaking of which, if you’re not familiar with Conway’s Game of Life, the rules are as follows: The goal of this post is to train a neural network to play Conway’s Game of Life without explicitly teaching it the rules of the game.
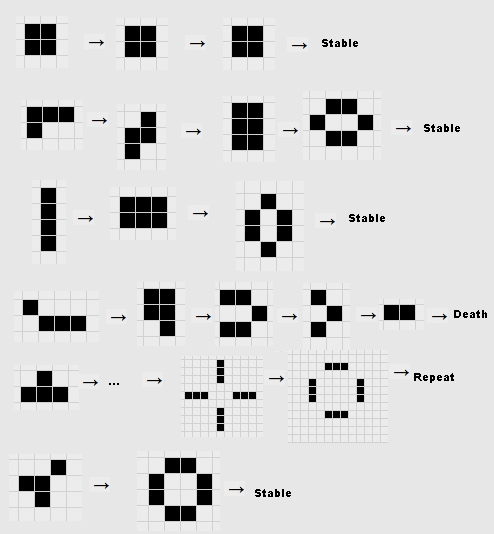
Related Posts Loading Unlabeled Images with ImageDataGenerator flow_from_directory in Keras Training/Validation Split with ImageDataGenerator in Keras Transfer Learning and Retraining Inception/MobileNet with TensorFlow and Docker
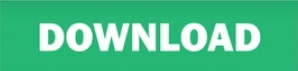